Artificial intelligence has revolutionized many fields, including image generation. With tools like DALL-E and Stable Diffusion, creating images from text prompts has become a fascinating reality. But how exactly do these AI image generators work? In this article, we’ll explore the underlying technologies and processes that enable AI to generate stunning images from simple text descriptions.
The Evolution of Image Generation: From GANs to Diffusion Models
Before diving into the intricacies of modern diffusion models, it’s important to understand the traditional method of generating images using Generative Adversarial Networks (GANs). GANs consist of two neural networks: a generator and a discriminator. The generator creates images, while the discriminator evaluates them, deciding if they are real or fake. Through this adversarial process, GANs improve their ability to create realistic images.

However, GANs have limitations, such as being hard to train and prone to issues like mode collapse, where the generator produces the same or very similar images repeatedly. These challenges led researchers to explore alternative methods, resulting in the development of diffusion models, which have since become a new standard for AI image generation.
Understanding Diffusion Models
Diffusion models simplify the image generation process by gradually adding and then removing noise from an image. The process starts with a clear image, to which noise is progressively added in small steps until it becomes indistinguishable from random noise. The AI then reverses this process, removing the noise in small steps to reconstruct the original image.
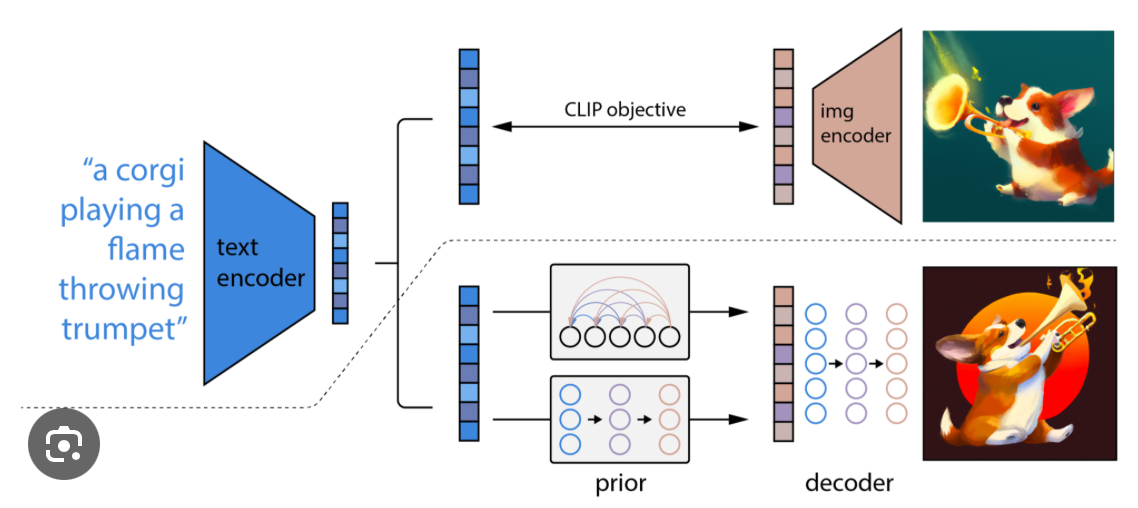
The key advantage of diffusion models is their iterative approach, which makes the task of generating high-quality images more manageable. Unlike GANs, which attempt to generate an image in one step, diffusion models break the process down into smaller, more controlled steps, leading to better and more stable results.
Training and Fine-Tuning Diffusion Models
Training a diffusion model involves feeding it millions or even billions of images, adding random noise to these images, and then training the model to predict and remove the noise. This process allows the AI to learn how to reconstruct an image from its noisy version, improving its ability to generate images over time.
The model’s performance is further enhanced by using a noise schedule—a method of controlling how much noise is added at each step.
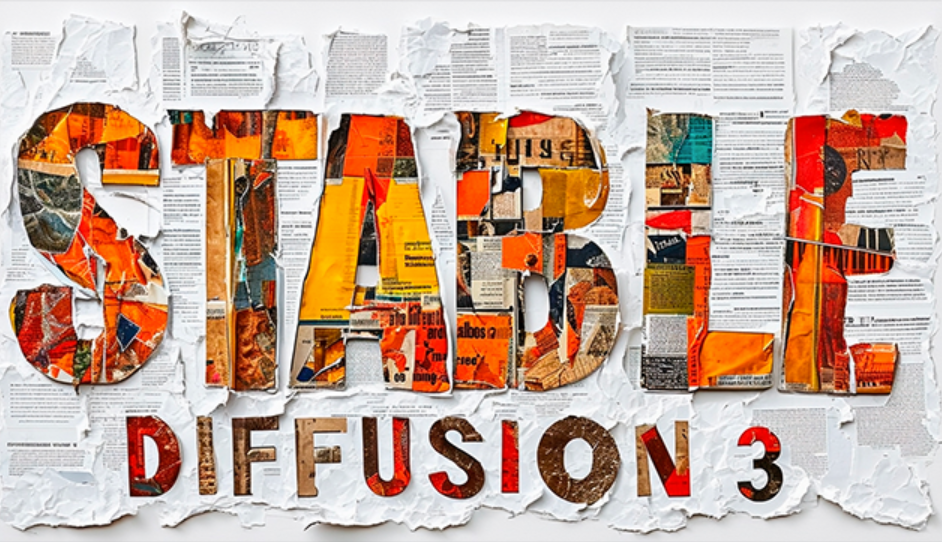
By varying the amount of noise and the number of steps, researchers can fine-tune the model to produce more accurate and visually pleasing results.
Guided Image Generation: Making AI Understand Prompts
One of the most exciting aspects of modern AI image generators is their ability to create images based on text prompts. This is achieved through a technique called classifier-free guidance, where the AI is trained to understand the relationship between text and images. The AI takes a text prompt, such as “a frog on stilts,” and uses it to guide the image generation process, ensuring that the final image aligns with the provided description.
To further refine the output, the AI runs the generation process twice—once with the text prompt and once without. By comparing the two results and amplifying the differences, the AI can produce images that closely match the user’s request. This method has significantly improved the quality and relevance of AI-generated images, making tools like DALL-E and Stable Diffusion more powerful and user-friendly.
Conclusion
AI image generation has come a long way, from the early days of GANs to the sophisticated diffusion models used today. These advancements have made it possible for anyone to generate high-quality images from simple text prompts, opening up new possibilities for creativity and innovation. As the Generative AI technology continues to evolve, we can expect even more impressive and accessible tools for creating AI-generated art.
Read more related articles in our Blog.